Riyasat Ohib
Georgia Institute of Technology. Ph.D. Candidate
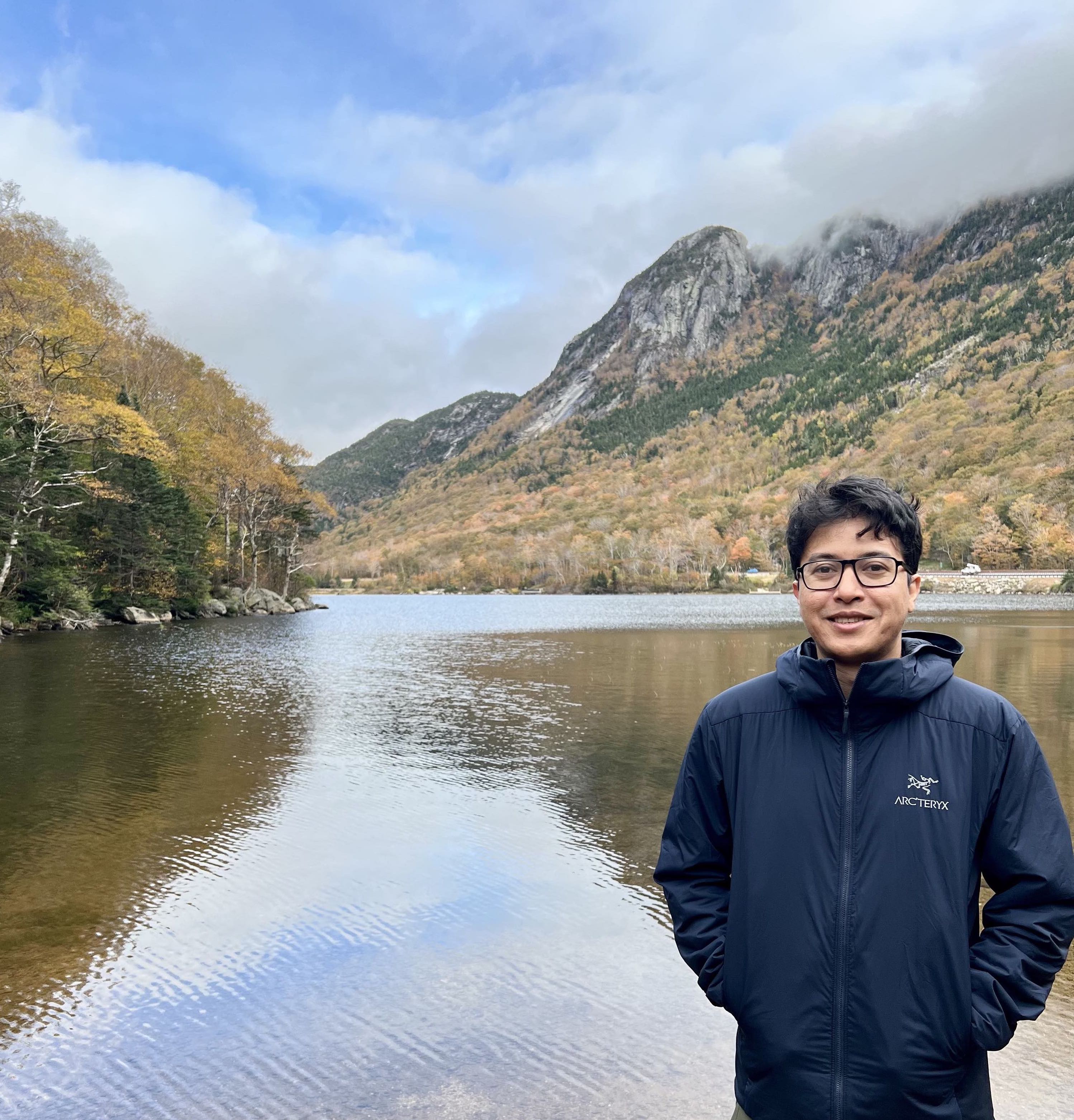
I am a Graduate Student in the Department of Electrical and Computer Engineering at the Georgia Institute of Technology. While I have a broad interest in learning algorithms, my current research primarily centers on the development of sparse and efficient neural networks and understanding the intricacies of their training process. I currently work as a Graduate Research Assistant at the Center for Translational Research in Neuro-imaging and Data Science (TReNDS), a joint research center by Georgia Tech, Georgia State and Emory University under the supervision of Dr. Vince Calhoun and Dr. Sergey Plis.
I am primarily interested in sparsity in deep learning, model compression, efficient AI and sparse learning. Beyond the traditional efficiency benefits of sparsity, I’m drawn to its potential in yielding models that not only outperform their dense counterparts but may also exhibit enhanced robustness and other favorable attributes often overlooked in conventional sparsity research. I am also involved in the areas of federated, multi-task and multimodal learning.
news
Mar 05, 2023 | Preliminary work accepted in ICLR 2023 Sparse Neural Networks workshop on communication efficient federated learning. Details coming soon!! |
---|---|
Oct 31, 2022 | Our work, Explicit Group Sparse Projection with Applications to Deep Learning and NMF has been published in the Transactions on Machine Learning Research (TMLR). Available at: OpenReview |
May 09, 2022 | Joined FAIR at Meta AI as a Research Scientist Intern to work on efficient ML and model sparsity research. My compression research library was integrated as part of the open source Fairscale library. |
Oct 20, 2021 | Our paper, “Single-Shot Pruning for Offline Reinforcement Learning” was accepted in Offline Reinforcement Learning Workshop, NeurIPS 2021. - Paper. |
Aug 27, 2021 | Started Ph.D. at Georgia Tech. I will be working on developing new techniques for sparse deep learning, with potential applications in federated, multi-task and multimodal learning. |